Examination Body In Kenya
The slips are unitless and the accelerations are in the unit of kph/s. Support Vector Machines SVMs, a sort of supervised machine learning algorithm, are generally used for classification and regression analysis. An SVM model, generally considered a non stochastic binary linear classifier, constructs an algorithm that allocates new instances to one of two categories for a set of data, each labelled as one category or the other. In an SVM model, instances are represented as points in a space domain, which are mapped for the instances of the separate groups to be divided by the widest gap. New instances are then mapped into the constructed space and categorized to be included in one of the two categories based on the established gap function. Although SVM models are normally applied to linear classification problems, they can also be applied to a nonlinear classification using the so called kernel trick that maps their input instances into high dimensional feature spaces . In this study, the Python language was used to build a linear SVM model with C value of one for identifying slippery roads. Deep learning, based on Artificial Neural Networks ANNs, is a sort of machine learning algorithm that utilizes multiple layers to analyze a high level of features from a set of examples. ANNs, inspired by information processing of biological brains, are computing systems with organized communication nodes. Among the learning methods are supervised, semi supervised, or unsupervised approaches. The term deep refers to the number of layers through which features are progressively extracted from the input.
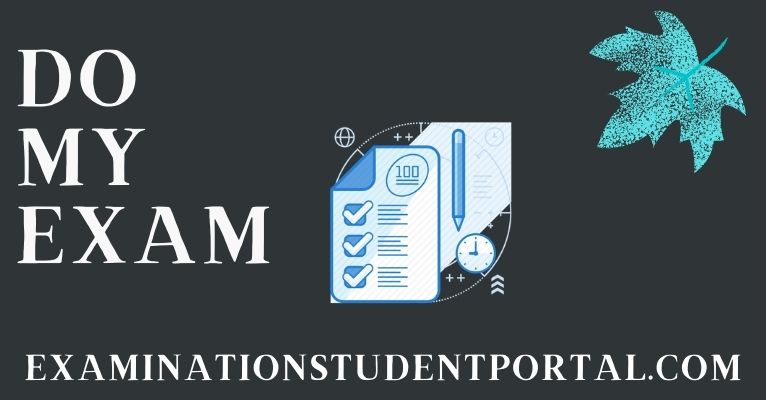
Animal University Courses Near Me
Our analysis extends the weekly return analysis in Karlsson, Loewenstein, and Seppi 2009 by including daily, weekly, and monthly returns and by allowing for nonlinearities, and our sample is much larger than in Gherzi et al. 2014. We generalize the ostrich effect to allow portfolio attention to depend on other market factors. Andries and Haddad 2014 predict that attention should decrease in volatility for loss averse investors. A related idea is that investors may emotionally disengage from the market in advance of periods in which they are worried about the risk of extreme outcomes. We call a negative correlation between attention and volatility the volatility ostrich effect. In contrast, trading motivated attention seems unlikely to decrease in market volatility. In addition, news media coverage of the stock market may stimulate financial attention by acting as a market wide analog to stock attention grabbing Barber and Odean 2008. Hypothesis 2: Attention decreases in market volatility. Hypothesis 3: Attention increases in news media coverage of the stock market. The second motivation for our investigation of attention is to understand the impact of attention on trading.
College Courses For Gas Engineer
This will be added to Academic Resources 2004 05 Internet MiniGuide. ZapMetaapMeta. com/ZapMeta is a meta search engine, a search tool that provide users the ability of simultaneously search multiple search engines under one interface. Meta search engines benefit users by saving them time and effort from having to individually visit multiple search engines in order to find the desired result. Along with web search, ZapMeta currently offer a directory based on data from The Open Directory Project and Product Search powered by Pricegrabber. Please refer to the Meta Search Engine FAQ to learn more about meta search engines.
Kenyatta University Art Courses
A. Cohen. But his defence of Marx involves a complete retreat to the mechanical interpretation of Kautsky and Plekhanov. Historically, however, there has always been a revolutionary alternative to either mechanical materialism or voluntarism. It existed in part even in the heyday of Kautskyism in some of the writings of Engels and in the work of the Italian Marxist, Labriola. But the need for a theoretical alternative did not become more widely apparent until the years of the First World War and the Russian Revolution proved the bankruptcy of Kautskyism.
Free Open University Courses Australia
Traditionally they have been compared, and competed, with LL parsers. So there is a similar analysis related to the number of lookahead tokens necessary to parse a language. An LRk parser can parse grammars that need k tokens of lookahead to be parsed. However LR grammars are less restrictive, and thus more powerful, than the corresponding LL grammars. For example, there is no need to exclude left recursive rules. Technically, LR grammars are a superset of LL grammars. One consequence of this is that you need only LR1 grammars, so usually the k is omitted. LR parsers are powerful and have great performance, so where is the catch?The tables they need are hard to build by hand and can grow very large for normal computer languages, so usually they are mostly used through parser generators. If you need to build a parser by hand, you would probably prefer a top down parser. Parser generators avoid the problem of creating such tables, but they do not solve the issue of the cost of generating and navigating such large tables. So there are simpler alternatives to the Canonical LR1 parser, described by Knuth.